Myriah Jaworski is a data privacy attorney, Melissa Ventrone a cybersecurity attorney and Eric Rouseau CIO and CSO at Clark Hill. Views are the authors’ own.
Imagine you’re on a video call with a major client. The client is going to share with you information regarding highly confidential and proprietary findings of a major study in the health care space. You shut your office door to make sure no one in the hallway can hear. You want to pay close attention and take notes. An alert from the videoconference platform pops up: “Enable video assistant.” You click on the link, which says the platform can take notes for you. It will summarize the meeting and draft an email to the client after the call thanking them for their time. Great, you think, as you turn the assistant on.
A week later, your IT team sends out a survey. One of the questions is whether you have used any artificial intelligence products at work over the last year. You know your company has a policy that requires you to get advance approval to use an AI tool. You click “No” and close the survey. But you have in fact used AI. You used it to record and process sensitive client data. That makes you part of an emerging challenge facing many employers: how to identify and address the use of shadow AI within their organization.
What is shadow AI?
Over the last decade, AI functionalities have been embedded into enterprise software platforms relied upon by many companies, often without enterprise users realizing it. For example, Office365 anti-phishing and email spam filters have leveraged AI and machine learning to improve detection since the mid-2000s, and cloud services offered by major providers include AI capabilities such as natural language processing, computer vision and recommendation systems since the mid-2000s as well. Yet, few organizations would identify themselves as using AI based on these features.
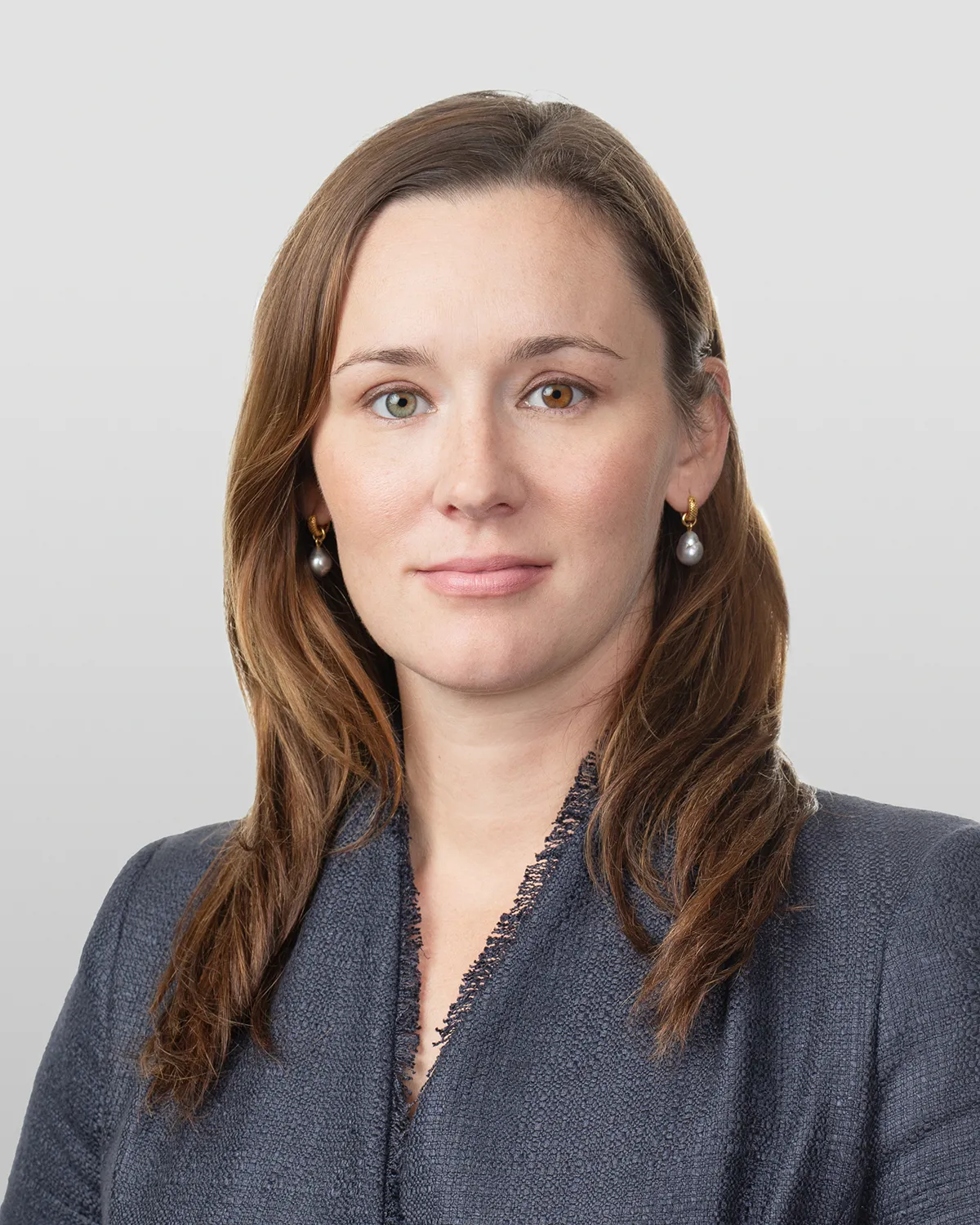
AI companies are more explicit about the AI capabilities of their tools today, but another problem has emerged: the use by employees of publicly available and often free AI products for work product, without disclosing the use to their employers, intentionally or not.
This “shadow AI” use occurs when employees use AI tools without the official knowledge, approval or oversight of the IT department or management.
It can be the result of AI use by individual employees or departments outside of a formal, centrally managed AI strategy. Shadow AI can also reflect the lack of awareness that a tool or technology is powered by AI.
Here are a few examples of shadow AI use:
AI integration in enterprise software. In some cases, businesses might not realize the extent of AI's underlying presence. Cloud computing is a good example of an AI-powered offering that is not fully recognized by many businesses.
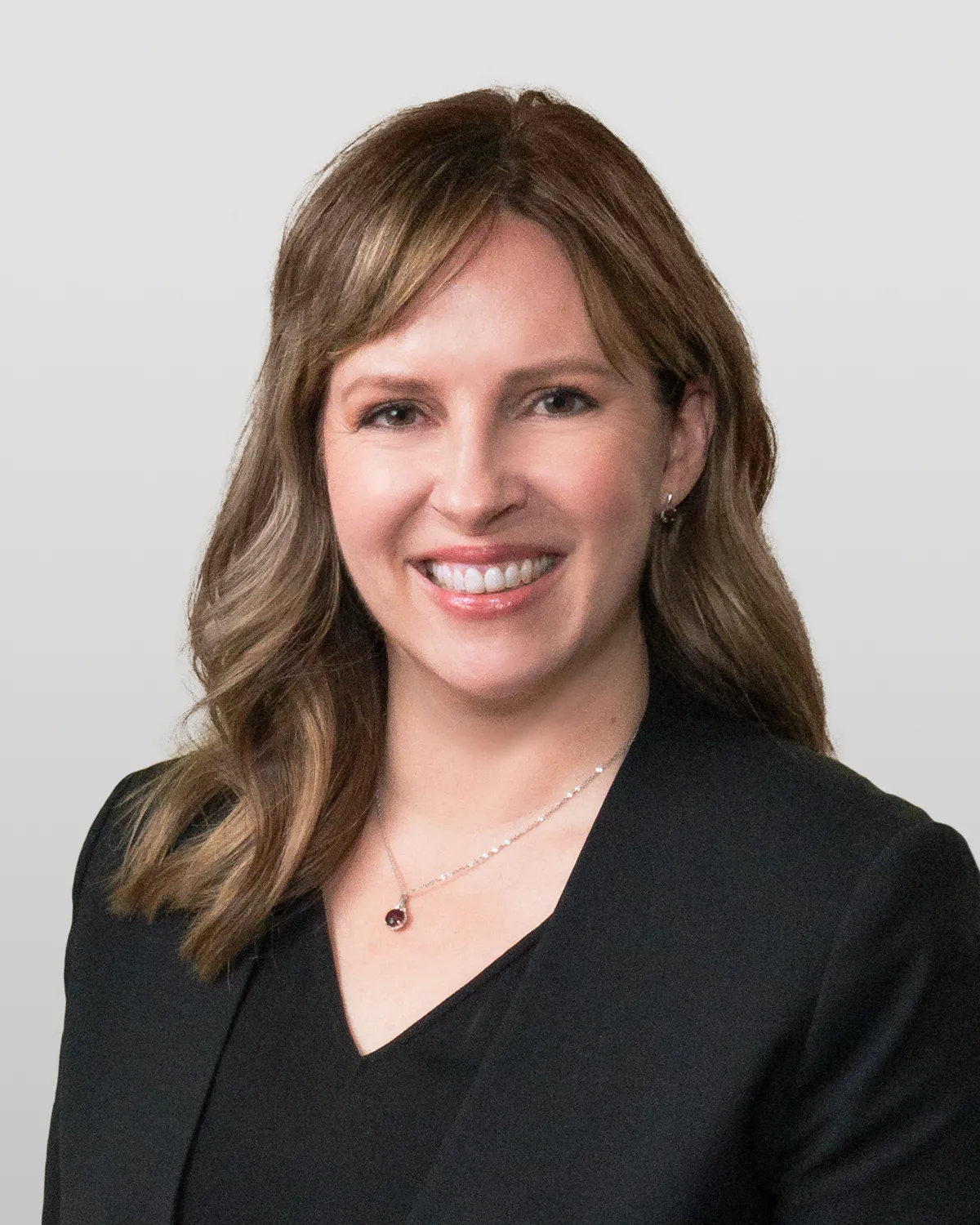
AI as a product feature. As in the videoconferencing example, AI can be a feature of a larger software product. In the case of videoconferencing, it was part of a product used for a non-AI purpose (videoconferencing). A document management system could be another instance. It might use AI to assist with content indexing and search optimization, but businesses typically know it as a document management tool.
AI enhanced hardware. Similar to software product features, hardware devices and equipment, such as cameras, sensors and IoT devices, can incorporate AI for image recognition, speech recognition or predictive maintenance. Organizations might use these devices without necessarily recognizing the AI components.
Unofficial implementation of AI tools by individuals or business units. In these instances, employees or business units understand that they are using AI tools, and choose to use them despite potentially running afoul of their organization's acceptable use guidance. The tools are ordinarily publicly available and, in many instances, free. ChatGPT, Llama and Bard are common examples used by individual employees. Real-world examples of individual employee use of publicly available AI tools exist in which the use resulted in the disclosure of confidential business information or proprietary client information. Often in these instances, employees either act without full understanding of the potential risks of the tools or they’re willing to disregard those risks in the belief the improvement they get out out of the tools is in the business’ best interest.
Addressing shadow AI use
Why is shadow AI a potential problem? Although its use presents risk, many organizations have subjected software and hardware to some form of third-party vendor management process which includes an evaluation of security and privacy risk, and contractual confidentiality promises. So, even where the AI computing is not fully understood, the solutions themselves have been deemed secure by the organization.
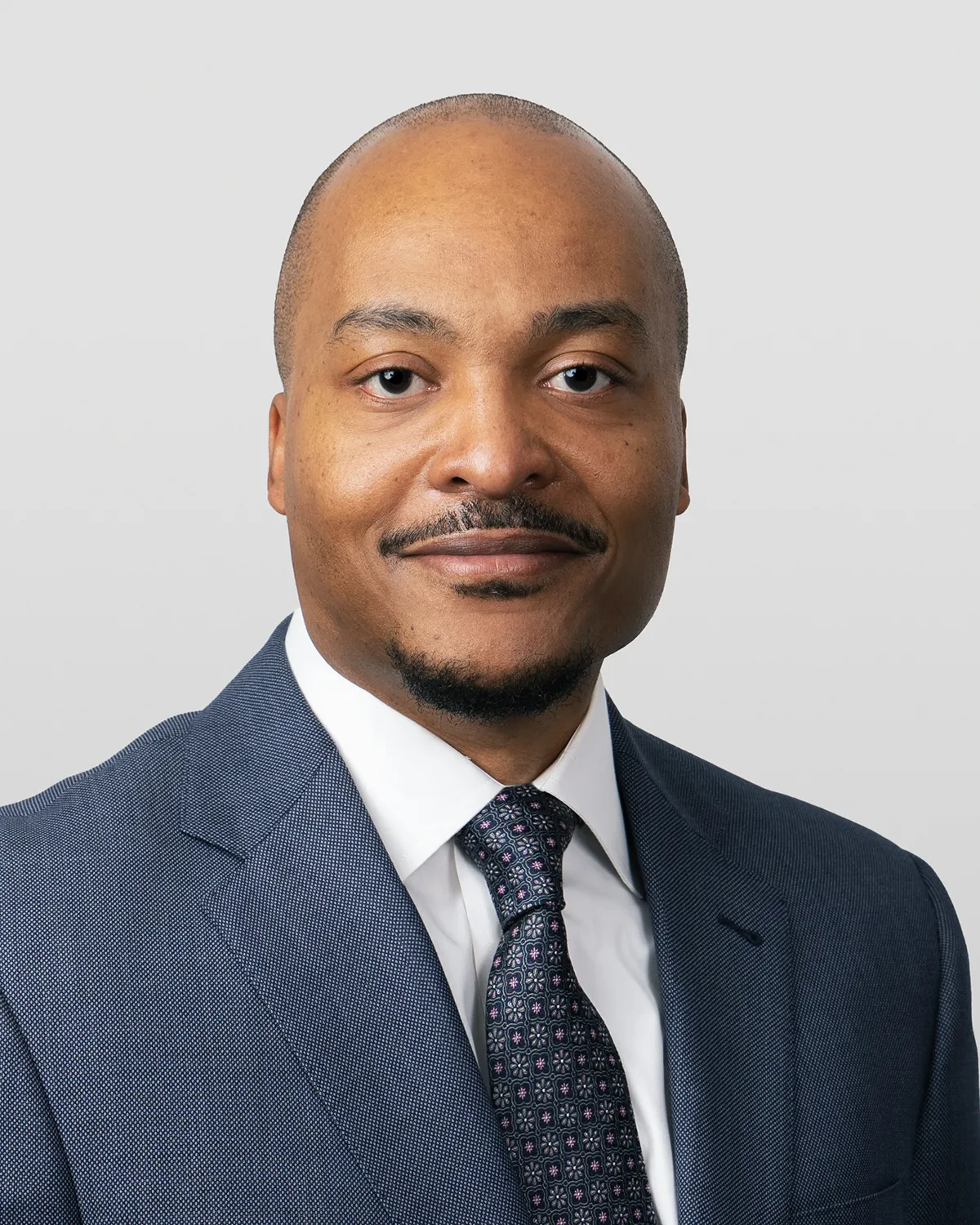
The same cannot be said of unofficial implementation or use of AI tools by employees or business units. That means it presents the most risk to an organization and must be prioritized in any shadow AI strategy. This is especially true where shadow AI tools are used to process confidential or proprietary company information, or information that is regulated under laws such as privacy, health care or financial laws.
Strategy components
There are a few must-have components to an effective shadow AI strategy.
Staff survey. Surveying staff can provide valuable insight into the extent of shadow AI use, while also providing a channel for employees to share feelings concerning AI adoption. To be effective, respondents should be told that their participation will not have an impact on their job tenure and the business should consider whether allowing respondents’ individual anonymity is appropriate.
A typical survey should look at AI use from a number of perspectives:
Usage. Ask employees if they use AI tools in their daily work. You should provide a list of common AI tools and leave space for respondents to specify the tools they use. A business should explicitly state that individual use of AI tools related to work should be disclosed in response to the survey.
Purpose. Inquire about the specific tasks or purposes for which AI tools are used. Are they using AI for data analysis, customer support, marketing or other functions?
Frequency. Determine how often employees use AI tools — daily, weekly, monthly, rarely or never.
Satisfaction. Ask about their satisfaction level with the AI tools they use.
Barriers. Inquire if there are any barriers or challenges that impede their use of AI tools.
You will also want to provide an open-ended opportunity for employees to share any comments related to AI tool usage.
Based on the survey results, develop an action plan to address any issues or opportunities identified. This might include improving AI training, addressing challenges or optimizing AI tool usage. Consider communicating the results of the survey with employees to demonstrate that their feedback is valued and to keep them informed about the organization's AI initiatives.
Acceptable use policy. Many organizations have developed a policy that sets forth the acceptable, restricted and prohibited uses of AI tools by employees. As with other company policies, an acceptable use policy is only as effective as company communication, training and enforcement. To this end, a company should clearly articulate its policy to all employees, contractors and stakeholders. This can be achieved through training sessions, dissemination of written guidelines and regular reminders as to the existence of the policy and its scope. Businesses should help employees understand the potential risks associated with non-compliance, and the company's commitment to responsible AI use.
Monitoring and auditing are essential components of policy enforcement, and the organization may implement technical controls to track AI system usage and data handling practices. These controls can include logging AI system activities, conducting regular audits and using AI monitoring tools to detect potential policy violations. Automated alerts and reporting mechanisms can be set up to notify relevant personnel when deviations from the policy occur, enabling swift intervention.
Consequences for policy violations should be clearly defined and consistently enforced. The AI acceptable use policy should outline the disciplinary actions that will be taken if employees or teams are found in breach of the policy. These consequences could range from warnings and retraining to more severe measures such as suspension, depending on the severity of the violation. Enforcing consequences sends a strong message about the organization’s commitment to responsible AI use and helps deter potential misconduct.
Technical controls. Businesses may be able to leverage existing technical controls to identify the use of shadow AI within their organization. For example, a business can establish network traffic monitoring systems or secure web gateways to detect data flows and application usage patterns. By analyzing this data, IT teams can pinpoint unexpected AI-related activities, websites and applications that might not be officially recognized or authorized by company policies.
Next, organizations can deploy endpoint detection and response solutions that focus on the devices and endpoints where shadow AI implementations may occur. EDR tools can identify unauthorized AI software installations, track their behavior and provide insights into potential security risks. What’s more, these tools can monitor for unusual file or process activity associated with shadow AI applications, helping organizations take prompt action to mitigate any potential threats or breaches.
An organization’s continuous vulnerability scanning solution and penetration testing activities can help proactively identify security weaknesses related to the use of shadow AI. These technical controls assess the security posture of the organization's systems and applications, including any unauthorized AI implementations. Regular testing can help uncover vulnerabilities and weaknesses that may be exploited by malicious actors or expose sensitive data.
And last, depending on the business risk profile, blocking of known external AI sites to prohibit access from company devices and networks may be appropriate.
By combining these technical controls, organizations can develop a comprehensive strategy to detect and manage shadow AI, ensuring that all AI initiatives align with security and compliance requirements while minimizing risks associated with unsanctioned AI deployments.
Reporting channels. A company may also consider leveraging internal reporting channels and whistleblower hotlines to detect and address the use of shadow AI within an organization. These mechanisms provide employees with confidential and safe means to report concerns or suspicions regarding the use of unauthorized AI tools or implementations. As when used for other purposes, employees should be educated about the existence of these channels and assured of the confidentiality and protection they offer. The reporting process should be straightforward, ensuring that employees can easily submit their concerns without fear of retaliation. Providing anonymous reporting options can further encourage employees to come forward with information about shadow AI use. Investigations should be conducted thoroughly, and if shadow AI is discovered, appropriate corrective actions should be implemented promptly.
Third-party vendor management protocols. Where Shadow AI is the result of embedding AI features into vendor software or products, updating existing third-party vendor management protocols can help. When onboarding third-party vendors or partners, organizations should include AI-related questions in their due diligence process, requiring the vendor to disclose if they AI is embedded in their product or a part of their service and provide details on each. This information will help organizations identify potential shadow AI risks early in the vendor relationship. Once AI use is identified, more difficult questions may emerge which can be addressed through the vendor contracting process. These issues include data ownership of AI outputs and models, audits of AI outputs, how to measure effectiveness of AI models at issue and indemnification/liability for AI defects or harms.
Strategy integration
Of course, shadow AI detection should one part of a larger AI governance strategy within an organization. An effective strategy helps businesses navigate the complex and emerging landscape of AI regulatory landscape, while also working to evaluate responsible, ethical and compliant use of AI technologies.
Such a strategy will typically include the creation of a cross-functional AI stakeholder team or committee responsible for AI governance.
This team should be comprised of members from various departments, each with distinct roles and responsibilities that collectively contribute to a comprehensive AI governance framework:
Executive leadership. The team should include senior executives or members of the C-suite who provide strategic direction and buy-in for AI governance efforts. They set the overall vision and priorities, allocate resources and ensure that AI aligns with the organization's strategic goals.
Legal and compliance experts. Legal professionals are crucial for navigating the complex regulatory landscape of AI. They are responsible for ensuring that AI initiatives comply with relevant laws and regulations, such as employee/HR laws, data privacy requirements, intellectual property, and industry-specific regulations.
Data stewards and data scientists. Data stewards oversee data quality, integrity and access. Data scientists contribute their expertise in data analysis, model development and model validation. They collaborate to ensure that data used for AI is accurate, representative and ethically sourced.
Ethics and diversity specialists. Depending on the business use of AI, the team may include an ethics or diversity specialist to focus on issues of fairness, bias mitigation, transparency and accountability. This stakeholder would work to ensure that AI systems do not discriminate against any group and that ethical principles are embedded throughout the AI lifecycle.
IT and security professionals. IT experts play a critical role in securing AI systems, protecting data and implementing technical controls. They ensure that AI applications are resilient to cyber threats and that AI assets are adequately protected.
Risk management and audit teams. These professionals assess and manage risks associated with AI, conduct regular audits of AI systems and provide recommendations for improvement. They help the organization stay vigilant against potential issues and liabilities.
Together, the AI team or committee should establish AI policies, oversee the monitoring of the deployment of AI tools, evaluate related risks and recommend adjustments to AI implementations. In addition to the roll out an AI use policy, the AI stakeholder committee should work to communicate the company policy internally to staff and raise awareness about the use of shadow AI within the organization.
In an era where AI is increasingly integrated into vendor software and business operations, recognizing the existence of AI systems operating outside the purview of official oversight is an important business priority. Through a combination of comprehensive technical controls, robust reporting mechanisms and a culture of transparency, organizations can shine a light on shadow AI and mitigate the risks of unsanctioned AI usage.