Jonathan Koch is an appellate attorney and shareholder at Smith Haughey Rice & Roegge. Views are the author’s own.
The Nevada federal district court decision in May that rejected claims of price-fixing through the use of algorithmic tools raises questions about the strength of the Department of Justice’s antitrust case against RealPage, the company whose software apartment management companies use to help them set rental rates. It also raise questions about other antitrust claims that have arisen against artificial intelligence-based software companies over the last several years.
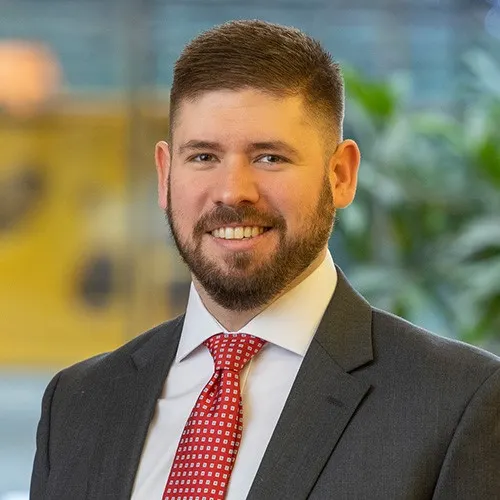
In the Nevada case, Gibson v. Cendyn Group LLC, the district court dismissed a class action suit for price fixing hotel rooms. In the decision, the court held that using common pricing algorithms alone does not equate to antitrust violations. By dismissing the case, the decision represents a skepticism that commutative AI technology necessarily implicates antitrust claims.
The district court considered if Cendyn, and a number of Las Vegas Strip hotel operators, violated Section 1 of the Sherman Antitrust Act by engaging in an unlawful conspiracy through their use of pricing software, which allegedly inflated hotel room prices through a hub-and-spoke agreement.
Broadly, Section 1 of the Sherman Antitrust Act prohibits contracts, combinations or conspiracies in restraint of trade. A “hub-and-spoke” conspiracy involves a central player (the hub) coordinating an agreement among other parties (the spokes), with a “rim” of coordination between the spokes. To prove a violation, plaintiffs must plausibly allege that the defendants engaged in anticompetitive behavior, either through explicit or tacit agreements that unreasonably restrained trade, typically involving an exchange of confidential information or uniformity in pricing decisions.
Gibson argued that Cendyn entered into a hub-and-spoke conspiracy by using Cendyn’s pricing software, which recommended pricing decisions that allegedly led to inflated room rates. However, the court found several key deficiencies in the plaintiffs’ claims:
- No evidence of agreement: The court found that Gibson failed to plausibly allege that the hotel defendants entered into any tacit or explicit agreement to follow Cendyn’s pricing recommendations. The software allowed hotel operators to accept or override pricing recommendations, which undermined the claim of a coordinated price-fixing conspiracy. The court noted that simply using the same software does not by itself indicate a violation of the Sherman Act.
- Timing of software use: The hotel defendants began using the software over a ten-year period, making it implausible that there was a coordinated effort or simultaneous adoption of the software for price-fixing purposes. The court distinguished this case from precedents where competitors adopted the same pricing strategies in close temporal proximity, which could suggest collusion.
- No exchange of confidential information: Plaintiffs did not adequately allege that the hotels exchanged any non-public or confidential pricing information through the software. Instead, the software incorporated publicly available pricing data, which is not a violation of antitrust law.
- No obligation to accept pricing recommendations: The court emphasized that the hotels were not obligated to accept Cendyn’s pricing recommendations and frequently overrode them. This flexibility in pricing decisions indicated independent, rather than coordinated, behavior among the hotels.
As a result, the court ruled in favor of the defendants, granting their motion to dismiss the case with prejudice.
Application of Gibson to RealPage
In RealPage, the DOJ similarly alleges that the company’s algorithmic pricing software used by landlords and property managers facilitates collusion, leading to inflated rental prices. The DOJ contends that RealPage’s software allows landlords to coordinate rent increases, reducing competition and prioritizing profit over market-driven pricing.
Three facts from Gibson suggest structural weaknesses in the DOJ’s suit against RealPage.
First, just like in Gibson, the landlords using RealPage’s pricing software are likely to have adopted the platform at different times, undermining the theory that there was a simultaneous agreement to fix prices. The Gibson court emphasized that staggered adoption weakens any inference of collusion because it suggests independent decision-making rather than coordination or collusion. In the case of RealPage, unless the DOJ can prove that all landlords subscribed to the platform simultaneously or in close proximity, the claim of collusion is weak to nonexistent.
Second, in Gibson, the plaintiffs failed to show that hotel defendants exchanged confidential information through the software. Similarly, unless RealPage’s platform enables landlords to share non-public pricing information between themselves, it is unclear how the software could even theoretically facilitate illegal price-fixing. If RealPage only uses publicly available data or anonymized information — which is common with AI-based software — it is analogous to the Gibson decision, where the court held that incorporating public data into pricing decisions doesn’t violate the Sherman Act.
Third, a key point in Gibson was that the hotel defendants were not obligated to accept the software’s pricing recommendations. The court found that because the hotels could override or reject the recommendations, the software was merely a tool for independent decision-making rather than a mechanism for enforcing collusive price uniformity. In RealPage’s case, if landlords are free to reject or override the pricing suggestions made by the software, then this autonomy seriously undercuts the argument that RealPage is enforcing a coordinated pricing scheme. Similar to Gibson, RealPage’s algorithmic suggestions would be just that — optional suggestions that landlords could choose to adopt at will, making it implausible to infer an illegal agreement.
As a result, RealPage’s use of pricing algorithms does not necessarily amount to an antitrust violation under Section 1 of the Sherman Act. Without evidence of simultaneous adoption of the software, exchanges of confidential information between users, or a binding agreement to follow the pricing recommendations, the DOJ’s case lacks the necessary elements to prove a price-fixing conspiracy. So, the DOJ’s RealPage case is likely to meet the same end as the case in Gibson.
Impact of Gibson on AI industry
Because courts have been hesitant to equate the use of common pricing algorithms with illegal price-fixing conspiracies, algorithmic pricing has gained widespread acceptance across many industries, including airlines, hotels, energy providers and even government-sponsored enterprises.
Airlines use dynamic pricing to adjust ticket prices based on factors like booking time, seat availability and demand patterns. This ensures that ticket prices reflect real-time market conditions and allows for efficient use of resources, optimizing both revenue for airlines and access for passengers.
Travel agencies implement algorithmic pricing to dynamically adjust rates for hotel rooms, flights and car rentals based on market trends and user search activity — which, unlike allegations made against such methods, fosters competition among service providers and ensures consumers have access to competitive rates.
Likewise, sports tickets marketplaces like Ticketmaster and StubHub employ dynamic pricing based on demand, event popularity, and seat location to benefit consumers by capturing the true market value of seats while ensuring that high-demand events are efficiently priced.
Even the government employs dynamic AI systems. Toll roads like E-ZPass, SunPass or FasTrack use dynamic toll pricing to ensure efficient road use and manage congestion by adjusting prices based on real-time traffic conditions and demand.
In sum, as more and more industries — including government agencies — become reliant on AI-driven pricing models, the outcome of RealPage and subsequent court cases will shape how AI technology is both used and regulated in competitive markets.