Bradley Merrill Thompson is member of the firm at Epstein Becker Green and Michael Shumpert is managing director at Mosaic Data Science. Views are the authors’ own.
General counsel are aware of the long and growing list of stories: An employment screening tool that doesn’t account for accents. Facial recognition software that struggles with darker skin tones. A selection app that shows a preference for certain backgrounds, education, or experience.
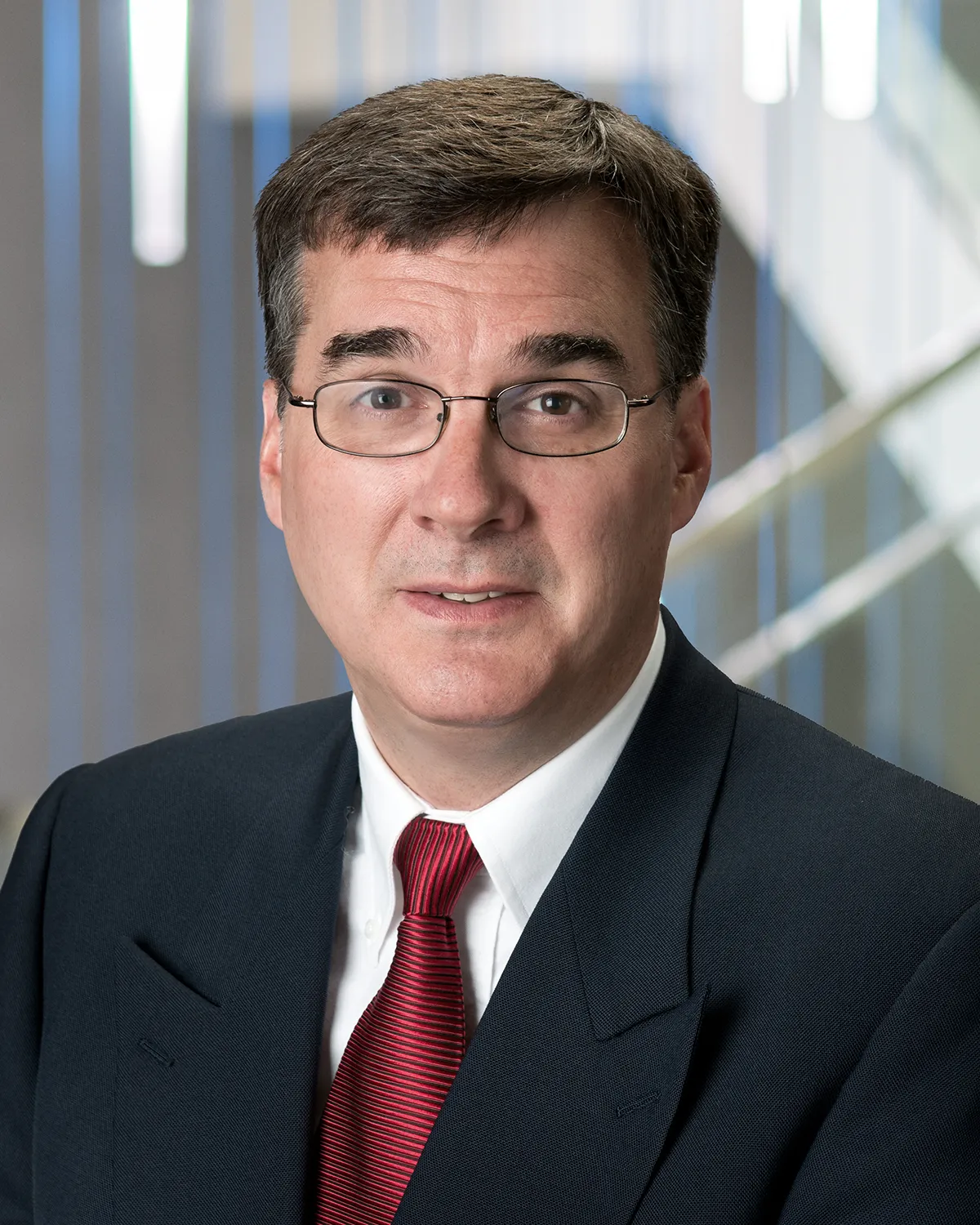
As local, state and federal agencies race to implement regulations to address these issues, general counsel face potentially costly legal liabilities and significant reputational harm if their organization attempts to use new AI-powered technologies in their hiring.
Can’t data scientists just “fix it”? Isn’t there a simple change to the math involved that could address the error?
Unfortunately, the answer is no, in many cases. There are two intertwined layers to the complexity that make remediation a matter of judgment requiring expert knowledge in data science and law. Those layers are (1) the technical challenges around finding and correcting bias, and (2) the legal complexities around defining what bias is and when it is acceptable.
Technical obstacles
Bias can creep into algorithmic decision-making in many ways, including by training an algorithm on data that contains encoded prior human bias, failing to ensure that the training data includes adequate representation of smaller groups, assumptions or mistakes made in the design and coding of the algorithm and measurement bias where the data collected for training differ from that collected in the real world, to name just a few.
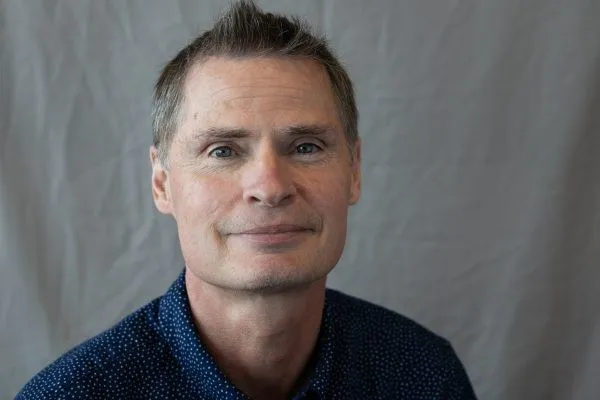
Can we overcome those problems simply by making sure that the data analyzed by the algorithm excludes data on sensitive attributes like age, sex and race? Unfortunately, sensitive information can be inferred from other information—information that has value that we do not want to lose.
You would think at least finding discrimination in an algorithm would be easy, but no. A key part in testing algorithms is having a metric to determine whether unlawful discrimination exists. But we don’t even have a uniformly agreed upon measure of what constitutes bias in, for example, qualitative outputs from large language models.
When bias is found, often it cannot simply be eradicated. Unless it is based on finding new, suitable data to supplement the existing training set, improving equality in the overall output often means reducing overall accuracy.
Fighting algorithmic bias
Given those technical challenges, there are judgments that need to be made, and this is where the law comes into play.
An example of these challenges and how the law needs to guide decision-making is an AI system that aids in predicting the risk of heart disease: This model would likely be trained on patient record datasets, including factors such as age, sex, race, ethnicity and medical history. What if the dataset is biased, such as it skewing heavily to white males between the ages of 50 and 60? The results from the model likely will predict that those patients are more at risk of heart disease, while other patients that don’t fall in that demographic are less likely to have heart disease despite the truth.
There are several areas where data scientists and attorneys need to work together to ensure that the AI model is not biased. Among them:
- Adhering to existing regulatory AI frameworks (for health, FDA, HIPPA, NIST, etc.). This will help to mitigate the risk of legal liability and build trust with patients and the public.
- Ensuring the data are high quality and representative of the population the model will serve.
- For training purposes, the data scientists want to use readily available data. Still, the company also knows that readily available data don’t completely represent all the possible patients. What data are necessary for a robust AI model?
- Exactly which demographic categories need to be evaluated? Which laws are implicated, and which protected categories must be tested?
- If training reveals gaps in performance for certain groups, does the law allow those deficiencies to be addressed through some sort of remediation, such as truthful labeling or secondary human intervention, or does the model have to be retrained on data that might be hard to obtain?
- Implementing fairness constraints: These constraints can help ensure the model does not discriminate against any group of people.
- There are multiple different fairness constraints that range from individual fairness to group fairness. Which one is appropriate?
- What is good enough in terms of the performance of each individual subpopulation?
- When unfairness is found, how do you fix it? Which trade-offs are acceptable? Can you mathematically adjust?
- What about software that works well in the hands of the developers but then produces biased results when used in a new population? Regulators are making it clear that because there can be differences between the data on which a model is trained and ultimately used, some users have an affirmative legal obligation to ensure that the model does not behave in a biased manner once it is in the users’ hands.
- Addressing bias over time:
- New York, other states, and the federal government are moving toward requiring periodic auditing of the systems for bias. General counsel need to work with data scientists to develop an audit program that meets the requirements of those laws.
Conclusion
In some ways, it is easier to find certain types of discrimination committed by an algorithm than it would be if we were auditing a purely human decision. But even though all the software code and data are right before our eyes, evaluating the performance of these models is difficult at best. We cannot achieve perfection, but collaboration between data scientists and attorneys is the key to developing algorithms that are compliant with anti-discrimination laws.